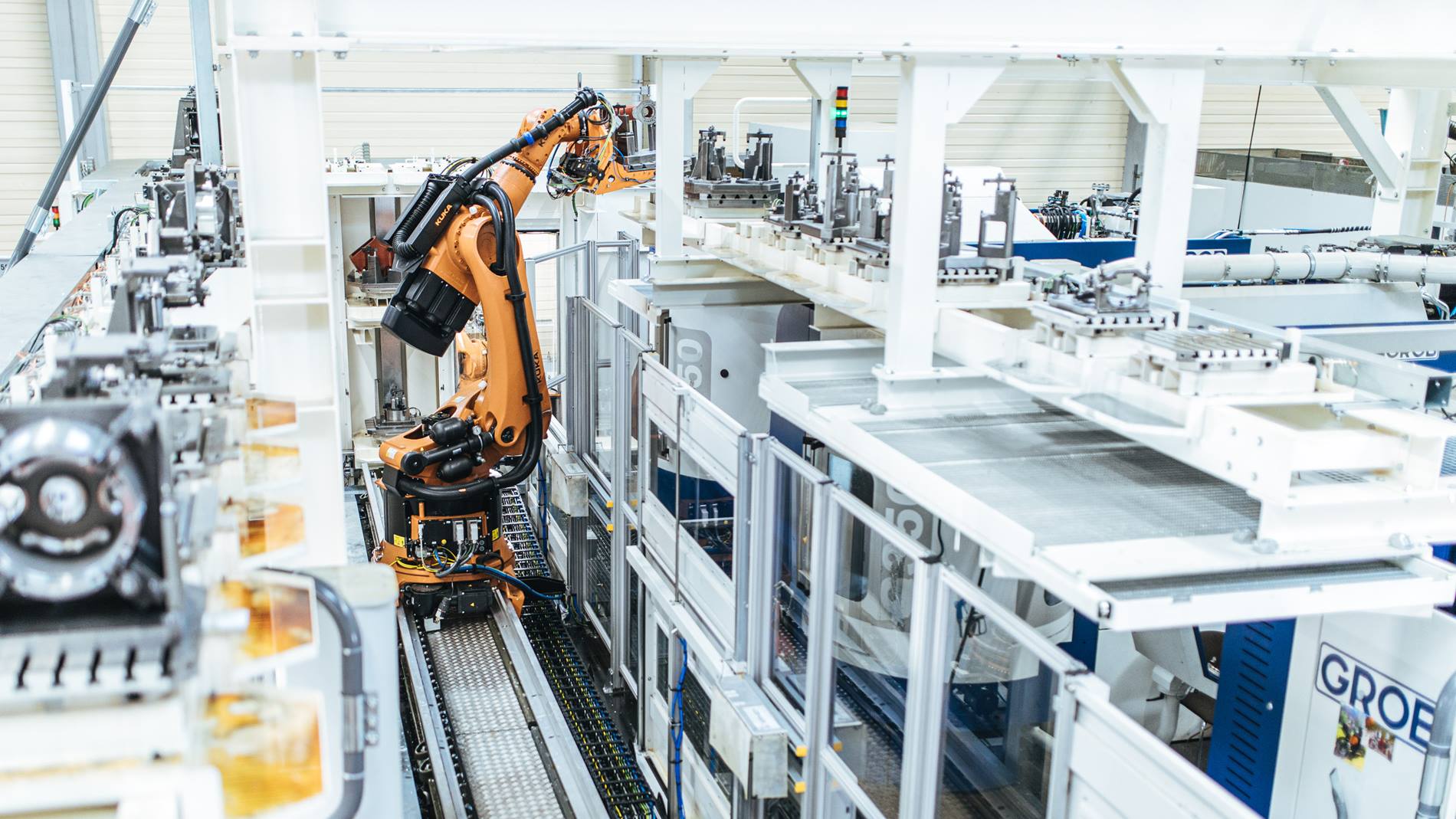
plus10 software tools get the most out of existing fill & finish lines at Schwarzkopf
+ 17.4% throughput increase identified by data-based plus10 optimization software. On large, fully automated and therefore confusing fill & finish lines at Schwarzkopf & Henkel, Shannon® and Darwin proved to be particularly beneficial.
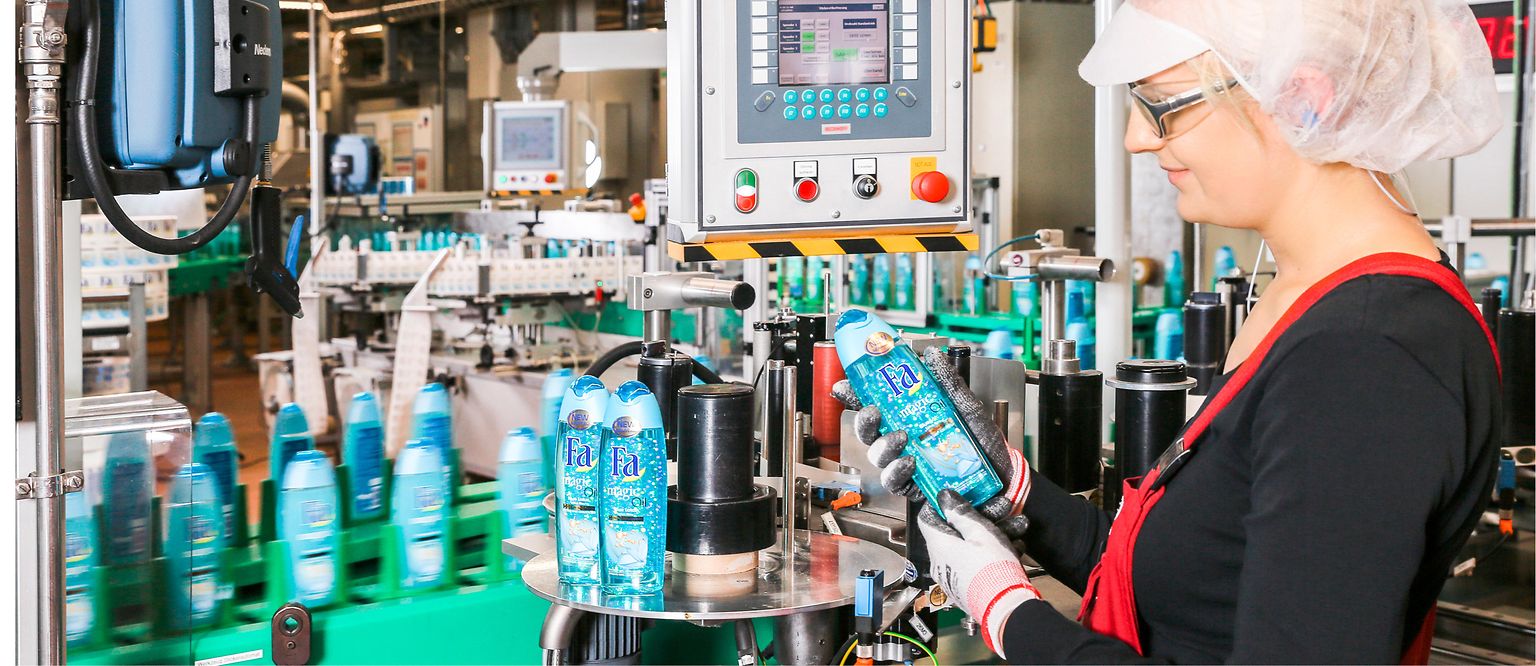
Short stops, technical faults and cycle time losses in highly automated production plants reduce their overall equipment effectiveness (OEE). To counteract this in the best possible way, plus10 offers data-based software tools that not only identify causal malfunctions and performance losses, but also directly provide concrete optimization suggestions. Shannon® communicates with operators via smartphone, tablet or smartwatch, forming a supportive worker assistance with situational suggestions in real time in case of malfunctions or upcoming activities of the next minutes, such as material shortage of a certain packaging material. Darwin also provides full transparency of cycle times at station level and visualizes the signal history of involved signals of relevant machine components in real time.
Combined use of Shannon® and Darwin especially for highly automated fill & finish production lines
In both the GMP-regulated pharmaceutical sector and the non-GMP consumer goods sector, the following applies: Particularly in large and thus complex highly automated fill & finish production lines that run around the clock in multi-shift operation, plus10 with Shannon® offers a significant advantage, since in the event of a machine stop, the operating personnel are referred directly to the location of a malfunction, e.g. a specific servo axis in a station, and are provided with concrete solution suggestions. As a result, troubleshooting times can be eliminated and downtime reduced, which means higher productivity for the entire plant. Conversely, the stability of individual machine components or even implemented shutdown and conceptual optimization measures can be quantitatively evaluated over several weeks or months of operation.
Thanks to the continuous learning analysis of high-frequency machine data and the simultaneous collection of contextual knowledge from all employees, more and more cause and solution suggestions are available over time in the event of a malfunction. Step-by-step instructions can also be used to train new and less experienced operators using the Shannon® app. By reducing short stops and outages, Shannon® enables a significant and automatically measured improvement in Mean-Time-To-Repair (MTTR) across all shifts.
If operations are running smoothly, Darwin, the Machine Performance Finder, provides additional functions to optimize the performance of the line in addition to technical availability. The evaluation of the dynamic bottleneck of the entire line is available at the individual process level on the basis of a cycle time analysis, in order to quantitatively and verifiably initiate programming or design optimization measures and to be able to clearly evaluate their effectiveness after implementation, also using Darwin. In addition, with the Signal Viewer, Darwin offers the possibility to visualize and thus analyze relevant signals over time.
Concrete application: Shannon® and Darwin on a Fill & Finish line from Schwarzkopf & Henkel
Thanks to the high-frequency data connection of all machine controls of different types involved in the process via plus10 DataCollector and the implementation of the Shannon® and Darwin software tools, Schwarzkopf & Henkel was able to achieve significant optimization successes in terms of technical availability and system performance. A fully automated and interlinked filling and packaging line for consumer goods was considered, with a total of 12 process steps divided among several stations of different machine manufacturers, which were continuously linked with each other. The initial cycle time was 0.17 seconds. The biggest loss factors were short stops due to empty or full buffers and the resulting startup and shutdown loss times.
Already in the implementation phase, in which a variety of different PLCs of all generations and manufacturers were connected (Siemens, Beckhoff, Schneider Electric), the intelligent software tools provided process experts at the plant with important insights into plant behavior and technically detailed optimization potential. In this context, data-based transparency about occurring faults (number and duration per shift) could be created, which enabled more effective shift handover and root cause analysis. Based on this, the detailed signal behavior for signal and cycle time analysis was revealed in a data-based manner. Furthermore, Darwin offered several analysis options for experts, which additionally enabled optimization of cycle time and output.
In summary, Schwarzkopf was able to add significant value to its highly automated consumer goods production with Shannon® and Darwin:
- Detailed transparency of downtime per shift at station level for effective shift handover and basis for conceptual optimization
- Detailed transparency of cycle times at station level as a basis for conceptual optimization
- Faster root cause identification in the optimization process and during downtimes thanks to visualization of the signal behavior using the integrated plus10 signal viewer
A simulative scenario analysis based on the real plant behavior also provided decisive insights for evaluating alternative material flow designs for the plant. Here, it was possible to analyze how the total throughput of the plant would change in the event of real malfunction behavior of all individual processes if a correspondingly higher or lower number of buffer belts were installed at one point or if control logics for the material flow were changed. The simulation of the different scenarios regarding throughput and utilization could be carried out realistically on the basis of the machine control data made available by plus10 and thus learned behavior models.
The simulation results showed, among other things, that an increase in throughput of + 3.3% is possible by optimizing the number of workpiece carriers. Furthermore, potentials of up to + 4.5% could be identified by simulating the buffer scenarios. The greatest levers for increasing output are the adjustment of belt speeds (+ 16.9% throughput) and the influence of individual stations (+ 17.4% throughput). In general, various losses of different interactions of the interlinked system could be identified with the simulation based on the plus10 data and models.
Overall, it was shown that significant results and potentials are identified through the use and operation of the plus10 software tools Shannon® and Darwin. Or in the words of the responsible operating engineer Lutz Kaiser at Schwarzkopf:
"With the intelligent software from plus10, we were already able to benefit from detailed transparency about downtimes and process times during the implementation phase. The signal-based root cause analysis by the Signal Viewer as well as the detailed fault analysis on station level were particularly profitable. With the help of the simulation based on the data, it was possible to define a package of measures to increase output."
(Image: © 2020 Henkel AG & Co. KGaA. All rights reserved)
News from plus10.
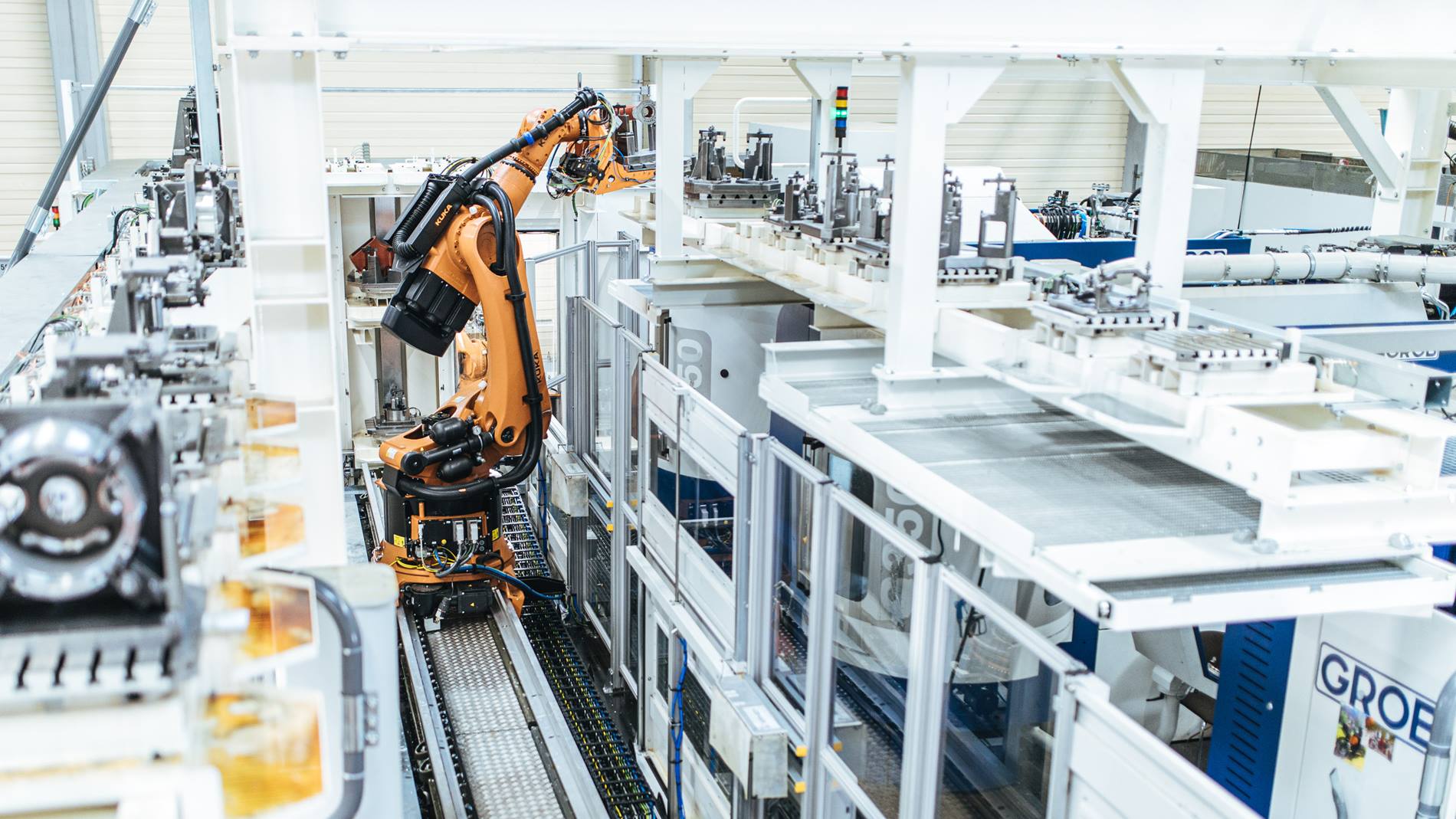

Microsoft Intelligent Manufacturing Award 2021 - plus10 and Haselmeier among winners
.webp)